Var3PPred: A Novel Tool to Predict Pathogenic Variants in Autoinflammatory Disorders
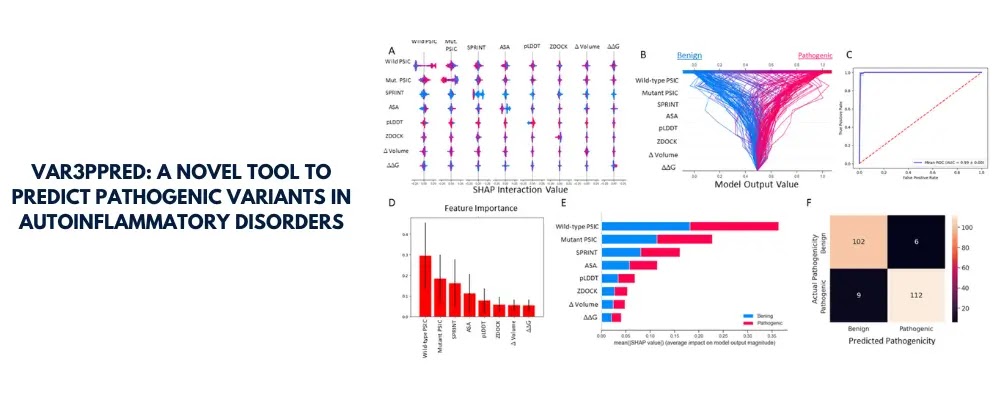
Identifying pathogenic variants in genes related to autoinflammatory disorders poses significant clinical challenges, particularly when dealing with variants of uncertain significance (VUS). These genetic alterations often exhibit subtle or ambiguous consequences that complicate their classification. To address this, our team has developed Var3PPred, a cutting-edge pathogenicity classifier designed to enhance the precision of variant classification in systemic autoinflammatory diseases (SAIDs) through a comprehensive integration of protein-protein interaction analysis and 3D structural information.
Methodology
Our methodology involved assembling a robust dataset of 702 missense disease-associated variants from 35 genes linked to SAIDs, derived from the Infevers database. We employed the Synthetic Minority Over-sampling Technique (SMOTE) to balance the dataset, which initially comprised 130 benign and 572 pathogenic variants.
We utilized 3D docking analysis of protein-protein interactions, leveraging data from the STRING and Intact databases, and incorporated ZDOCK and SPRINT values weighted by HGPEC gene rank scores. Further, the integration of sequential and structural features such as changes in folding free energies (ΔΔG), accessible surface area, volume, per residue local distance difference test (pLDDT) scores, and position-specific independent counts (PSIC) scores were computed using tools like PyRosetta and AlphaFold2 (AF2).
Our approach demonstrated through extensive hyperparameter tuning of six machine learning algorithms that the random forest classifier emerged as the most effective, achieving an area under the receiver operating characteristic curve (AUROC) of 99% on the test set.
The success of Var3PPred highlights the potential of integrating advanced computational techniques with traditional genetic analysis. Future research could expand upon this by incorporating a broader array of genetic variations and extending the model to other complex genetic diseases. Moreover, continuous updates to the training datasets and algorithms will be vital as new genetic data becomes available.
Conclusion
Var3PPred represents a significant advancement in the field of genetic diagnostics for autoinflammatory diseases, providing a powerful tool for researchers and clinicians to discern the pathogenicity of complex genetic variants. This tool not only enhances our understanding of genetic underpinnings in SAIDs but also paves the way for more personalized medicine approaches in the management of these conditions.
Reference:
Bülbül A, Timucin E, Timuçin AC, Sezerman OU, Tahir Turanli E. 2024. Var3PPred: variant prediction based on 3-D structure and sequence analyses of protein-protein interactions on autoinflammatory diseases. PeerJ 12:e17297 https://doi.org/10.7717/peerj.17297